The free tokens will continue until business improves
The SaaS-ification of AI continues, with OpenAI and Google ready to go full 2010s to get people on board. Plus, durable execution returns as a topic du jour.
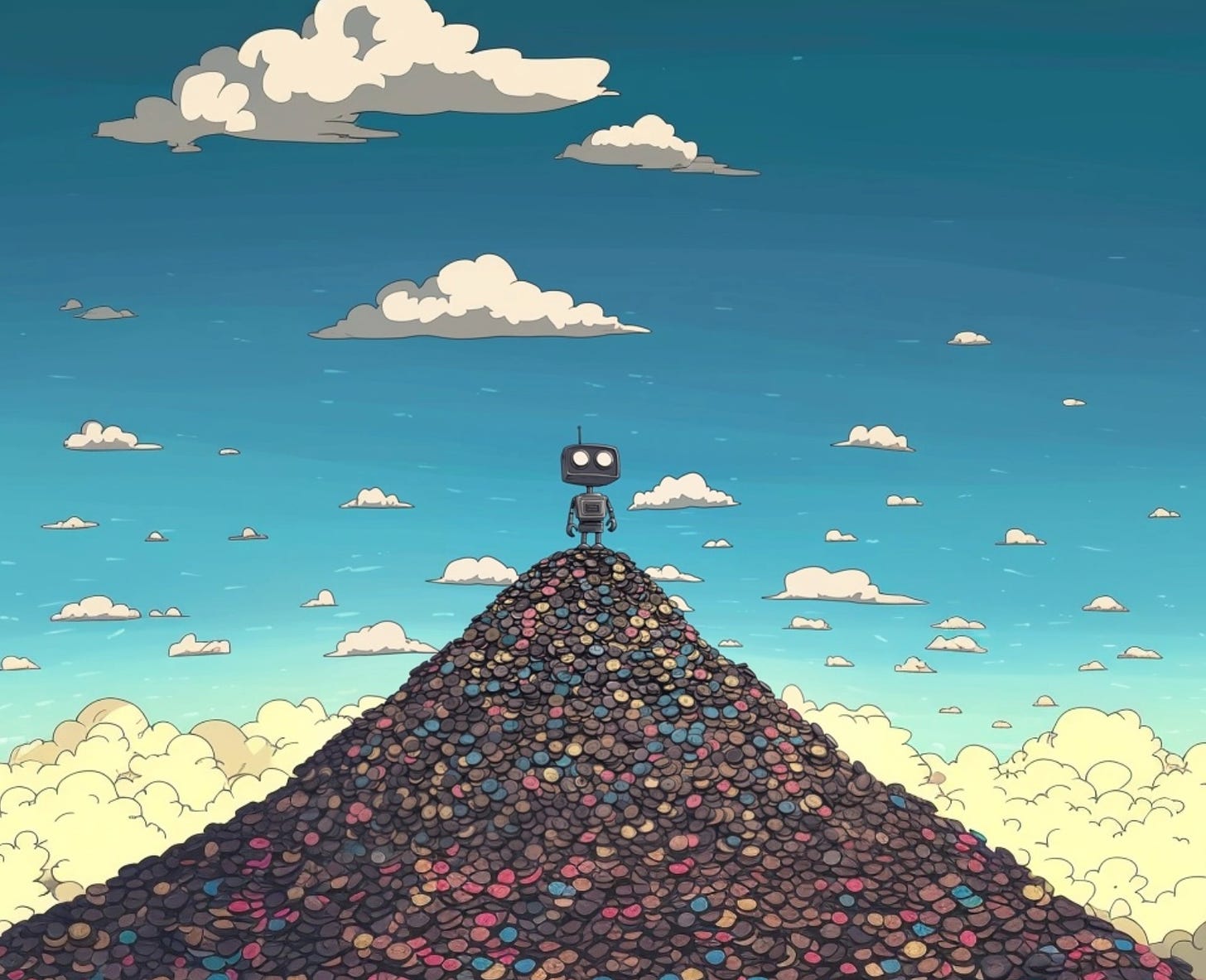
Two topics today: first is on all the free stuff we’re getting to convince me to fine-tune a model; second, why everyone is back to talking about durable execution. Once again, thank you everyone for your patience as I get ramped back up!
The free stuff playbook is back in AI
When Sam Altman was briefly removed from OpenAI in November last year, it represented this kind of weird moment in AI where most companies weren’t led with a very Silicon Valley growth-at-all-costs SaaS-ify everything mentality. Sure, there were a lot of mega-rounds, but one of OpenAI’s early advantages was that it was able to undercut the competition with an easy-to-use API.
Since then, the SaaS-ification of AI seems to have quickly become the norm. Providers are constantly racing each other to see how quickly they can send their prices as close to zero as possible to one-up each other. This is true with the more capable foundation models, but it’s also particularly true for the workhorse models from those model providers—the ones that benefit the most from customization on proprietary data.
And while the basic APIs are easy to simply swap amongst each other—whether that’s Google, Anthropic, OpenAI, or an inference platform like Together AI, that you can literally drop in with a few lines of code—the “lock-in” now potentially comes in an alternate form: fine-tuning APIs using proprietary data.
With fine-tuned models, which are essentially modified to perform well on specific use cases for your business like summarizing documents, the switching cost to a new service isn’t zero. Rather, the switching cost is both the time, headache, and tokens necessary to re-do a model customization job on a different service. And, just like in the on-demand and Web 2.0 boom, the two largest providers are trying to snag you with a bunch of free stuff.
OpenAI this week said it was making fine-tuning available for its GPT-4o. And while fine-tuning for GPT-4o mini has been available since late July to its higher-tier organizations, it’s now available for all paid usage tiers, per the blog post they put out. OpenAI already made 2 million training tokens available per day for GPT-4o mini, and this week it was making 1 million training tokens available per day for GPT-4o. Those free tokens are available through the next month, but also we know exactly how the price wars for Uber, DoorDash, Lyft, and others went with what seemed like endless subsidization. (Sure, that was ZIRP-era, but this time around we have GPU credits too.)
This is just a little less than two weeks after Google announced it was making its Gemini Flash 1.5 tuning product available for all developers. Gemini Flash 1.5, in addition to being very cheap, also hands out 1.5 billion free tokens per day to developers. Per Google’s pricing page, the tuning price for Gemini Flash is free of charge. Google even offers a free tier for its Gemini Pro 1.5, though it’s considerably lower volume at 1.6 million tokens per day at a much more limited rate (and per its pricing page tuning is unavailable). But the point is Google (and OpenAI) for now can afford this in the first place in the hopes of hosting the portfolios of custom models.
And while these pay-as-you-go models aren’t exactly what massive enterprises would adopt—that would more likely be provisioned usage and contracts with much more aggressive service level agreements—they serve as an easy and very aggressive onboard to getting stuff into production that can serve as a very enticing carrot for further usage.
It feels like the industry is quickly coalescing around a handful of companies with the resources to produce these kinds of powerful fine-tuned workhorse models—and they’re all trying to one-up each other in how much of a product they can put in front of companies to try to lock them into an ecosystem. We essentially saw all this before, in multiple times like the on-demand era, with companies giving away as much free stuff as they possibly could to eventually convert it to a sustainable business at some point.
And these more workhorse-focused fine-tuned models are essentially one of the end-games for enterprise focused tasks. Achieving some benefit in fine tuning for tasks like classification or summarization, in the case of Gemini, generally requires a number of examples in the low 100s.
But once you’ve collected those models, you can’t move them—OpenAI has them, and if you want to move them, you’ll have to re-do the process all over again either through Gemini or some alternate service. This is one of the main selling points of fine-tuning open source models, as you can move around fine-tuned versions of, say, Llama 3.1 8B as needed because they can port to any infrastructure that can run that kind of model.
And indeed, that’s also part of the threat that Databricks and Snowflake pose to OpenAI (along with Google and company). If fine-tuning with proprietary data is the way to unlock value—like building a custom summarization tool for my sales calls—the button closest to the data with the most optionality is going to be the most valuable. OpenAI faces the uphill battle of courting enterprises with its API that already likely have accounts with all of these abstraction layers and hyperscalers in the first place.
While we haven’t seen what it looks like just yet, the next obvious player here will be whatever rabbit Anthropic pulls out of its hat with its next-generation workhorse model. Right now you can fine-tune Claude Haiku through Amazon Bedrock, but it is nowhere remotely close to as easy as it is for the pay-as-you-go API approach that both Google and OpenAI offer. But Anthropic also has the benefit of Amazon promoting it to AWS customers, where that data for fine tuning already sits for many companies.
It also doesn’t help OpenAI that Cursor, a more flexible AI-enabled IDE where you plug in any provider’s API key, has a lot of hype among developers and investors I talk to lately. I wrote about the company several months ago about how one of Cursor’s biggest advantages was that you could pop in any code generation API key, making OpenAI even more disposable if a new superior model comes out—either proprietary or open source. (Cursor’s parent company Anysphere announced it raised $60 million this week.)
This constant deluge of free stuff shouldn’t exactly surprise anyone because all these companies are staffed with the same type of executive that lived through the on-demand era. For OpenAI in particular, both Sam Altman and chief operating officer Brad Lightcap hail from Y Combinator, the storied accelerator that spawned both Instacart and Dropbox—companies that, among others, popularized a “growth first, business later” mindset. Meanwhile, though Google has seemed to put together a rather comprehensive stack for AI, it still feels a lot like the same old Google that has been shoving free ad credits into the face of anyone who decides to open a G Suite account.
The on-demand era, and really Web 2.0 broadly, was often affectionately dubbed “venture-funded capitalism,” and came to a halt down when all that free capital dried up. With the duel in on-demand, it was largely around a handful of moat-building exercises: brand affinity, collecting data, wooing drivers (who ended up running both anyway), and altering consumer behavior away from hailing a cab or calling for delivery. For these companies, the last one was really the only one that was truly successful as they all struggled to build out long-term sustainable businesses.