Lakes, catalogs, and Snowflake's full court press on AI
Snowflake is converging with its rival, Databricks, faster than ever before. And its new CEO is gearing up for a partial "pivot" into AI.
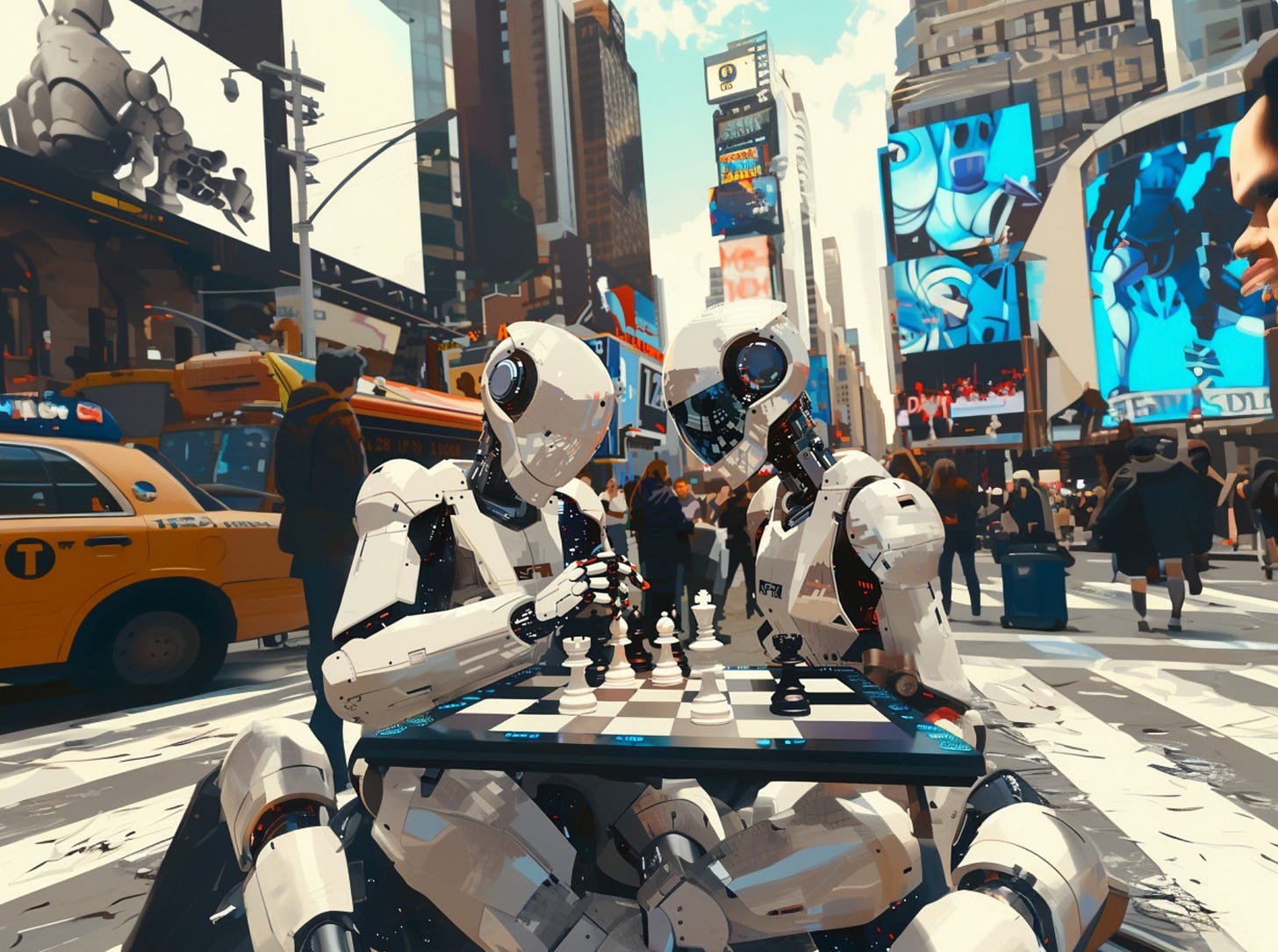
For the majority of its life, Snowflake was synonymous with analytics through its tools as as a data warehouse. It had made some exploratory moves into machine learning as it saw a new rival creeping up, including the abrupt $800 million acquisition of the Python developer platform Streamlit, which at the time had practically negligible revenue.
In the year and a half-ish since the launch of ChatGPT, a lot has changed. Snowflake has a new CEO, Sridhar Ramaswamy, through its $150 million acquisition of AI search startup Neeva. It’s announced a series of plays in AI with the launch of Snowflake Cortex last year. And this week at its Snowflake summit, its announcement largely sat in AI’s orbit. It has a new catalog tool (Polaris), a new observability suite (Snowflake Trail), a notebook tool, and many others that provide a heavy emphasis on AI.
What was a database company under its past series of leaders is increasingly a company focusing its efforts on building the developer and operational frameworks for AI. It’s not exactly a pivot—Snowflake is still the data warehouse company—but it it’s not… not a pivot.
People tell me that under its new CEO, Sridhar Ramaswamy, all hands on deck for AI is an understatement at Snowflake. A major sense of urgency that comes down from Ramaswamy—particularly the risk of missing the AI wave—is powering a lot of the extreme “build or buy” mentality in AI.
“[Snowflake’s new leadership] very, very genuinely believes—and for that matter, correctly, in my opinion—that we are essentially in a once in a generation technological inflection,” Adrian Treuille, head of Snowflake’s developer platform Streamlit that it acquired for $800 million, told me. “Five years ago, most machine learning researchers thought we were decades away from talking to decades away from talking to computers…. And now it's like, ChatGPT, yeah, no a problem. It's amazing. And so I would say that the the thinking about the importance of AI is is genuinely first principles.”
That’s materializing in a lot of forms, this week with the launch of a series of Iceberg- and AI-focused products. But Snowflake is also clearly in dealmaker mode, most recently evaluating Reka AI for a potential $1 billion per Bloomberg. A month prior to the deal petering out, Snowflake had launched its own large language model, Arctic, as well as a series of embeddings models. And while Databricks was the ultimate buyer for Tabular, an Iceberg storage platform, sources tell me Snowflake was aggressively in the mix for the 40-person startup going all the way back to April.
All this is pretty reasonable to expect for any company in the data abstraction layer among what are effectively considered among sources the three leaders: Snowflake, MongoDB, or Databricks. Enterprise data, which in many cases took years to get onto these platforms, is ripe for usage in modern AI. In fact, most of the value for AI is locked up in that data—either in the form of accessing it through retrieval augmented generation (RAG) or customizing the models directly with additional data.
While OpenAI’s fine-tuning APIs are incredibly straightforward to use, those same enterprises—especially ones with stricter privacy standards—aren’t likely to ship out their data to some API they don’t have direct control over. And that gives those data abstraction providers the ability to just add a customization or retrieval layer right on top of the data that enterprises have direct control over.
The difference here is that Snowflake, before the launch of ChatGPT and modern AI, was long considered a laggard in supporting machine learning. It didn’t support Python development until the launch of Snowpark in 2021. Databricks, meanwhile, had aggressively started in the opposite direction and then inched closer to Snowflake’s business over time.
But one of the biggest blockers to getting all these applications into production is having the suite of tooling around them—lineage, governance, development, observability, and to the extent possible, explainability. Snowflake, with its announcements this week, is bulldozing into all of those categories on its way to trying to be a backbone for AI development.
At its conference this week, Snowflake has effectively tried to brand itself as an “AI data cloud.” It’s a far cry from its summit in 2022 when one of its marquee announcements was Unistore, which was going after one of the holy grails of database technology in unifying analytical and transactional workloads.
Snowflake was long considered to be one of the key players in the abstraction layer, which has gone on to become a fundamental part of AI deployment. And with its launches it’s effectively growing into that role.
Lakes, warehouses, and another small startup worth more than $1 billion
Arguably the two most significant announcements from Snowflake this week was Polaris, a new open source catalog format built on Iceberg, and its suite of observability tools Snowflake Trail. Catalogs effectively serve as the governance layer of a data store, providing access and logging around data within an organization. And observability—basically the monitoring of performance of a given product—is emerging as a key requirement for many larger enterprises in order to graduate out of that proof-of-concept stage for AI.
Modern AI broadly is built around the premise of data lakes as a backbone for the abstraction layer. You take all unstructured data—PDFs, images, emails, transcripts, whatever—pre-process it into something more coherent and accessible, and shove it into a semi-accessible format. It’s also led to the birth of a whole chain of startups focused on piping that unstructured data into an unstructured data store like unstructured.io, Datavolo, Reducto, and others.
Snowflake and Databricks have also embraced competing formats for data lakes. While Databricks has its own format (that’s now open source) in Delta Lake, Snowflake effectively bet on the open source format Iceberg. Its announcements to date have all relied on Iceberg, which has proved to be an extremely popular file format.
While ongoing for several years now, Snowflake’s embrace of data lakes is somewhat of a break from its long singular focus on data warehouses. Founded in 2012, Snowflake pounced on the decline in the cost of cloud storage to build out what effectively remade the business intelligence and analytics layer and gave birth to a whole suite of tools to radically improve the quality of analytics. We generally refer to all these tools, including Dbt, Alation, Monte Carlo, and others, as the modern data stack.
As machine learning emerged as a much-larger-than-anticipated market with techniques and tools maturing on the cusp of the launch of ChatGPT, Snowflake pressed into the world of unstructured data—and data lakes. In late 2022, Snowflake launched Iceberg Tables, embracing the open source format that came out of Netflix four years prior. (Databricks, at the time, was already seeing usage of large language models in the form of BERT.)
Machine learning tooling built on top of data lakes, though, had long been Databricks’ sweet spot. Databricks announced the availability of Delta Lake in 2019, and in 2022 it announced that its 2.0 version of Delta Lake would be open source. And its 3.0 version, launched at last year’s summit, was also effectively a shot at a universal format for data lake management.
During all this time, Databricks has long tried to espouse the paradigm of a data lakehouse—a unified approach to data warehousing and lake management that allows for execution of all tasks, whether that’s business intelligence or machine learning. And Tabular, a storage platform built on Iceberg, was one of the best positioned tools to grow that paradigm.
The acquisition of Tabular, though, has been floated for weeks, going back to even April. The most recent number I had heard floated on the Snowflake side