What the suits said about AI this quarter
The fourth quarter of 2023 is (mostly) a wrap, and we have a fresh batch of tea leaves to over-analyze in AI.
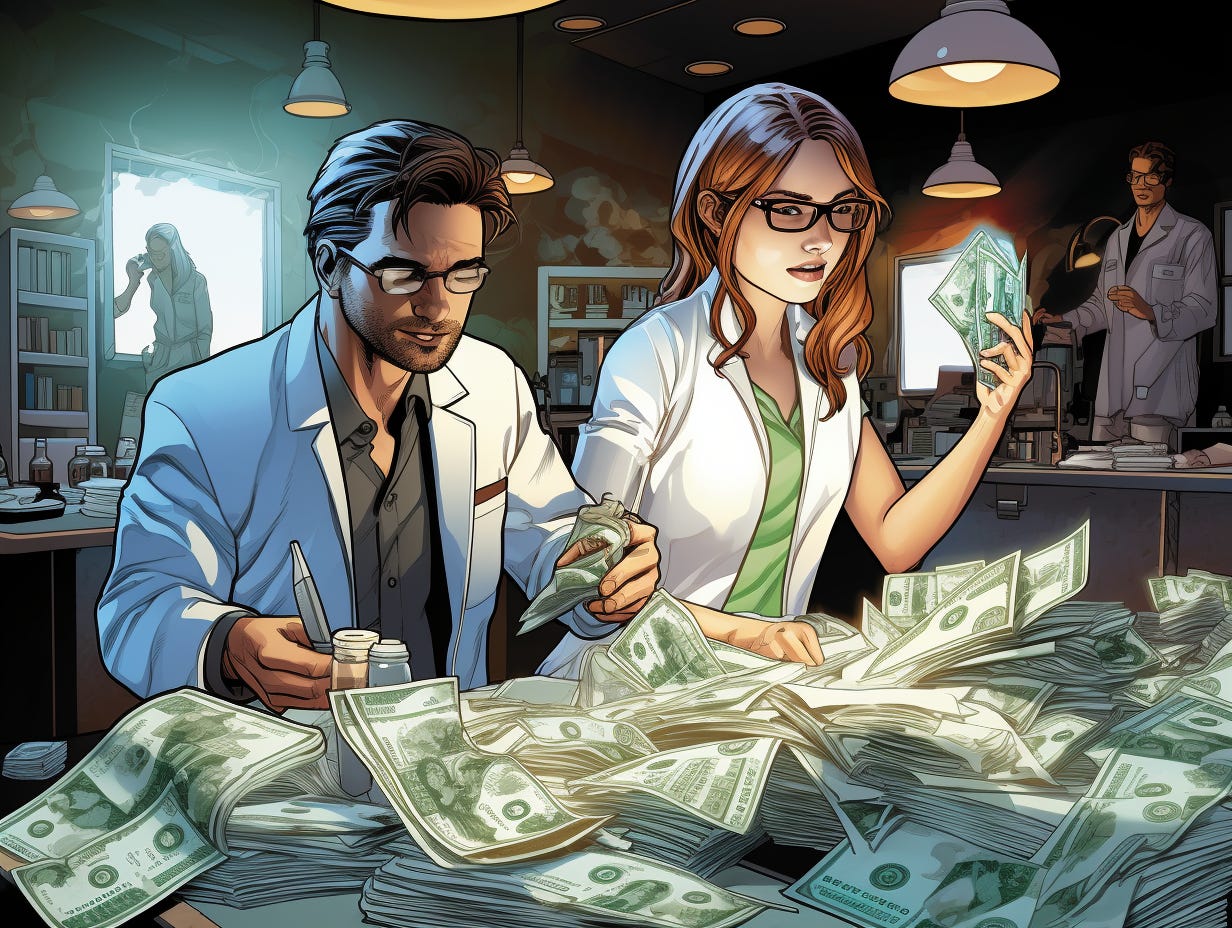
Supervised will be on a limited publishing schedule until 2/26 as part of a partial leave. Paid subscribers, including new ones up until 2/26, will received a comped week’s subscription. I’ll provide comped duration proportional to the missed number of issues (one week per every 2 issues) to existing paid subscribers when I resume a full publishing schedule in the last week of February.
Once a quarter, we get some nominally-direct insight into how the tech larger companies in the world are thinking strategically about their businesses through the probing of a bunch of banks grading their performance in the most near-term possible way: public stock price.
Usually this is relegated to core business metrics and other hullabaloo (remember traffic acquisition cost for Google, anyone?)—but since we’re flying into a world powered by a once-in-a-generation new technology, it seems everyone has to ask the AI Question these days. And, fortunately, this is pretty useful!
In general what we get from these kinds of AI questions are party lines of the subject matter. But in the context of an earnings report, there has to be at least a little more of a robust explanation for how it can Create A Lot Of Value For Shareholders. And now that we’re settling into the front-end of an actual business cycle for AI, we’re starting to get some of that.
More specifically, we got some additional context about the general arc of development in a few key areas:
Retrieval augmented generation: The hyperscalers (and by extension vector search providers) called out RAG as a part of general AI workflows. What started off as a bandaid to try to improve prompts has essentially transformed into a whole pillar of the chain of operations in these potential products. MongoDB (and Databricks to a certain extent) had already positioned itself as providing vector search as a core business, but it seems the eyes of the providers themselves are now fixed on RAG.
The flip to inference: There’s a whole meme around “we’ll be done training all this AI stuff at some point and everything will be inference.” Well, it turns out that could actually be a thing, based off some comments from Microsoft, but the expectation it seems is this is a kind of transient period.
The benefits of open source (and model gardens): Meta and Mistral have basically turned into the vanguards for open(ish) source models in AI. The general development and investment in open source software has always had tangible benefits for the core contributors, but that principle is indeed naturally extending into AI.
Uneven costs for AI: One note from Meta indicated that while the company is working on future models, the actual cost of those models might be variable due to the rapidly-developing nature of all of these techniques. What worked for Llama 2, for example, might not work for Llama 3 as Meta amasses a comically large cluster of Nvidia GPUs for its AI purposes.
Of course, the downside of these comments is they’re all considered “forward-looking statements” which have a permanent hedge that, hey, we might not actually do this or it might not be right, indicated at the beginning of every call in a Safe Harbor Statement (or the CYA, if you prefer).
So, as we think about all this quarterly earnings rigamarole, we have to kind of treat it as a tea leaf analysis. But any tea leaves are helpful in an industry that develops as quickly as AI! And startups in particular are looking for as many clues as possible about the strategies of all these larger companies they hope to partially disrupt. (The same holds for venture funds as well investing in all these startups.)
So let’s get into it:
The shift to inference (and local model opportunities)
One of the biggest criticisms of Databricks’ acquisition of MosaicML, which came in at $1.3 billion, was whether or not companies would move on from training as all their custom models and begin focusing all their efforts on using them in practice (as in, inferencing).
Well, it turns out it was quarter-ish right, but on a technicality. Microsoft, which setting aside its model garden is pretty much hosted OpenAI, suggested that the majority of AI workloads were focused on inferencing and fine-tuning, and not necessarily training.
Here’s what Microsoft CEO Satya Nadella said more specifically about that on the call:
Yeah, just on the inferencing and training, what you’re seeing, for the most part, is all inferencing. None of the large model training stuff is in any of our either numbers at all. Small bot batch training, so somebody doing finetuning or what have you, that will be there, but that’s sort of a minor part. Most of what you see in the Azure number is broadly inferencing.
And that makes sense! It’s been almost a year since the launch of GPT-4, and about six months since the launch of fine-tuning for GPT-3.5 Turbo. We’ve also had a little over six months of RAG being a thing, and Llama 2 came out a little more than six months ago (in a partnership with Microsoft). All the primitives were in place for companies to string together what they wanted their products to look like—as well as a number of startups enabling fine-tuning and hosting like Together AI—and we’re heading into a period where we’re going to see a lot of this in production.
But there are constant new developments in AI, with the foundation model providers putting an enormous amount of work into developing new ones—and companies with more specific needs potentially exploring their own pre-training. Nadella suggested this emerging train-then-inference-then-train type of “cycle” happening in AI.
Here’s more specifically what Nadella said about the topic: