The market for open source models is coming together
Mistral's latest model might spark an actual race to the bottom on cost. Plus, Intel's CEO Pat Gelsinger hates CUDA—does Intel actually have an answer to it?
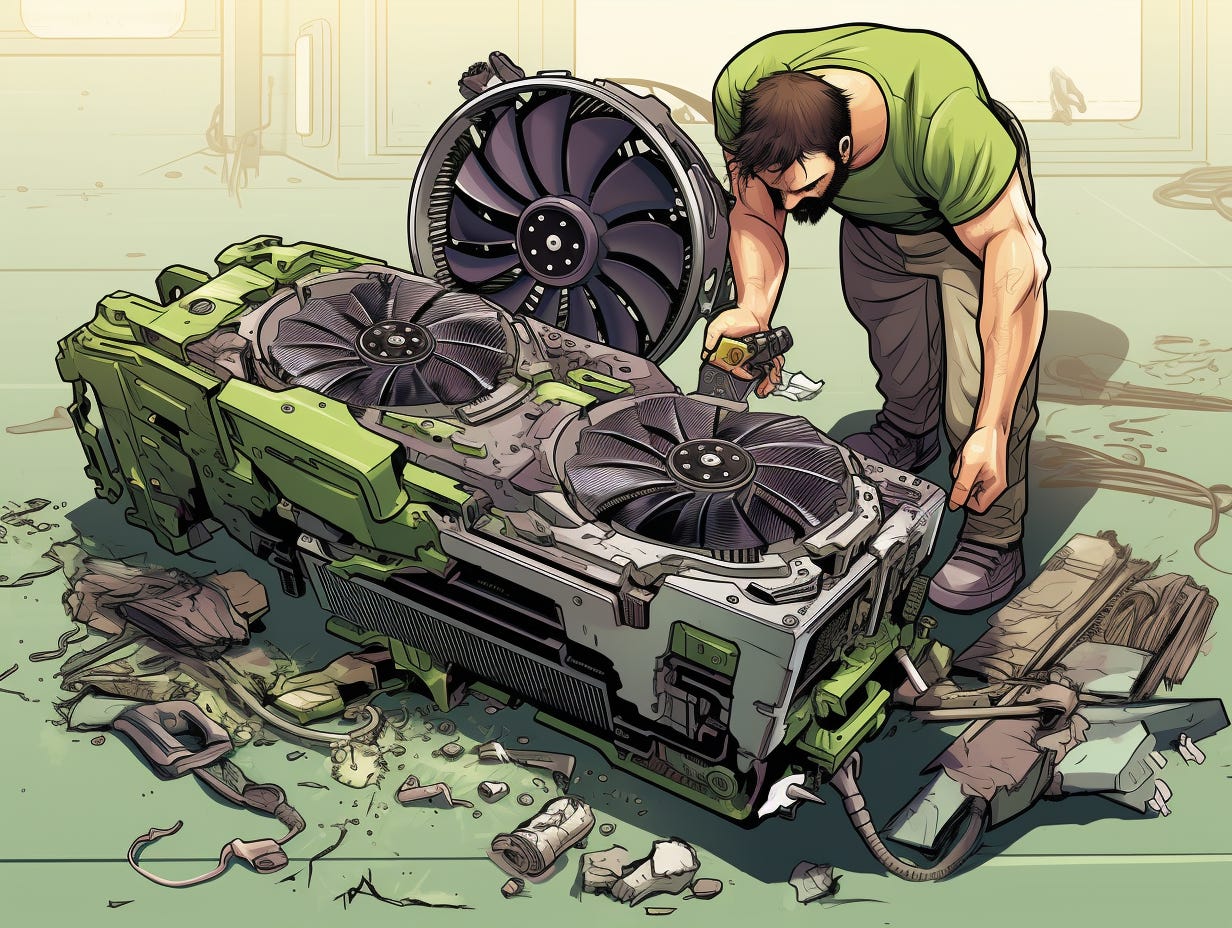
Double feature today! We’re talking about open source endpoints that are pretty cheap, and what a chip CEO said about Nvidia.
The business behind open source models takes shape
Okay! Some open source stuff happened in the past week. Specifically, we got an extremely cool and very good model from Mistral AI, a semi-mysterious Parisian startup that’s worth $2 billion even though it just launched its API access on Monday. Mistral’s new model, Mixtral, actually came out last week in extremely unceremonious fashion via a magnet link on the platform formerly known as Twitter.
So, let’s talk about the cost of all these models, and how we seem to be converging on that thing that we all expected to happen with the launch of GPT-4 and its open source cohort: the cost of pretty good models racing to the bottom.
Mixtral is a very good model that manages to, on some benchmarks, (barely) beat out Llama 70B and GPT 3.5-Turbo. The standard GPT 3.5-Turbo comparison benchmark is MMLU, which is essentially a multiple choice test, because that’s basically the best thing we got from OpenAI. This is important, because Mixtral is not a 70-billion parameter model, and instead architecture that combines a cluster of smaller models called a Mixture of Experts.
(I’m not going to go into a crazy amount of detail on the specifics of the model, because Nathan Lambert already has a ridiculously good teardown of the thing from a performance and technical perspective.)
This is the first landmark mixture of expert model to hit the open source community and due to its architecture, it’s able to run more efficiently than Llama 2 70B. Mixtral can even be run on a local device with oLlama or llama.cpp, though you need a considerable amount of memory. But MoEs (like GPT-4) offer an efficiency advantage where you can squeeze more performance out of a cluster of smaller models.
Here’s where things get a little weird: Mistral’s hosted version of Mixtral, as I read it from their pricing page, is expensive. And multiple other providers have started serving Mixtral at less than half the cost per million tokens. I did a quadruple-take on this because I am so concerned I am misreading it, so I’m just pulling this from their pricing page:
Here is how Mixtral on Mistral’s platform compares to some of the other options out there (including its closest equivalent, GPT 3.5-Turbo):
Together AI: $0.60 per million tokens.