Snowflake plunges into AI and OpenAI faces a new embedding challenger
Plus: Hugging Face seemingly gets closer to being a creator hub in AI.
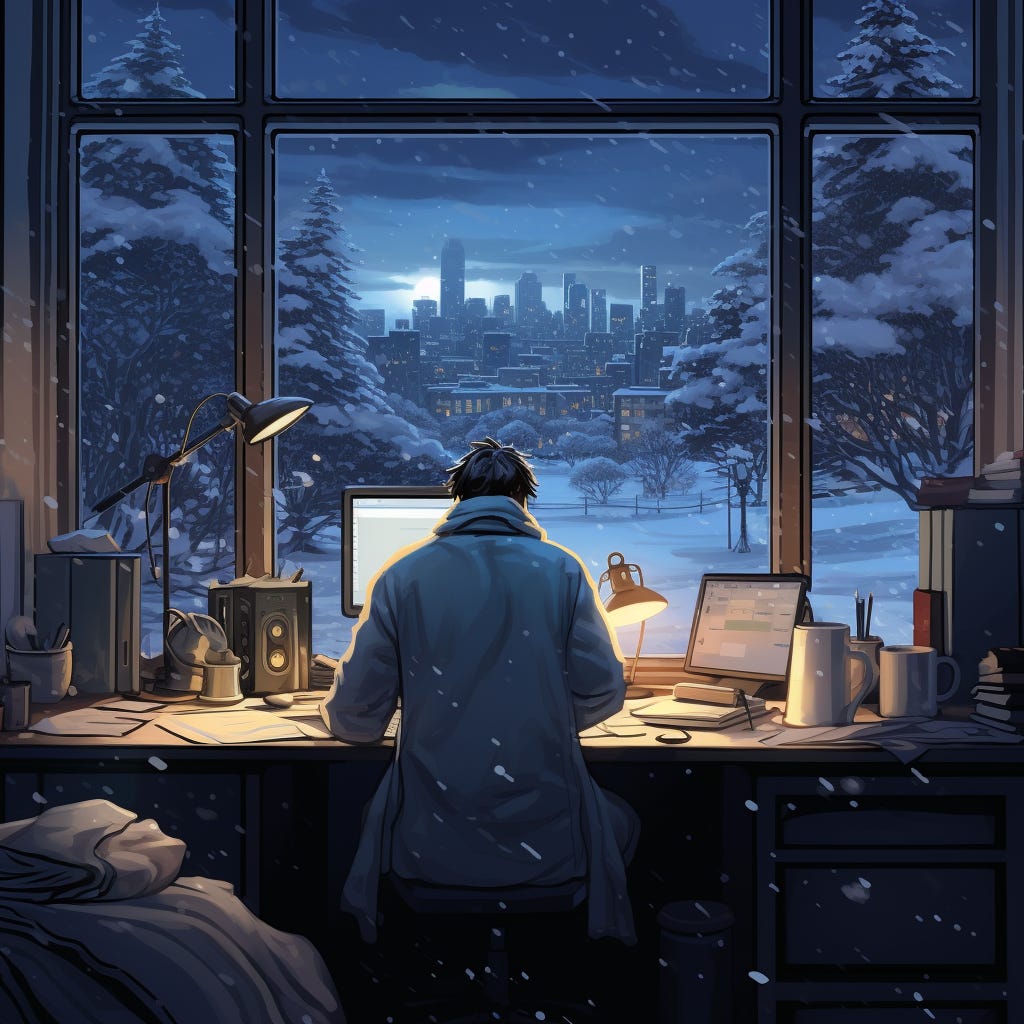
When Snowflake acquired Streamlit for $800 million early last year, machine learning was largely synonymous with deploying supervised and unsupervised learning models to accomplish a spectrum of business tasks—like predictive modeling for sales.
While the price tag was jaw-dropping for many, it seemed at the time like a kind of curious (though substantial) entry into data science and a way to grab a customer base that had largely been locked up by Databricks. Streamlit was effectively a way to create web interfaces for developers who lived and breathed cells and charts, and would spit out their coffee if someone told them to learn front-end web development and React.
With the advent of ChatGPT and modern large language models, “AI” means something a whole lot different than its flippant use to refer to fancy statistics in developer notebooks. And Snowflake—alongside its rival Databricks—are the primary home of the data for many companies that will make those language models useful in the first place.
While Snowflake showed a few early signs of movement in “modern” AI earlier this year at its summit with the release of Document AI, it’s making a much more substantial push this fall with the launch of a series of tools—particularly Cortex, announced this week.
Snowflake’s launches include a copilot, document AI, serverless functions for language model usage, and a few other natural drop-in places for language models. But perhaps the most interesting part of Cortex is a universal search that spans not only the data within Snowflake, but data across its entire sprawling ecosystem of native applications, data sets, and other components.
In Cortex’s universal search, we’re starting to see the emerging benefits from its acquisition of Neeva. Originally a search engine that stumbled in the consumer market, Neeva was effectively acquihired for $150 million earlier this year for its team and its technology. Sources tell me that getting Sridar Ramaswamy, a former Google lead, in the door was a big part of that deal.
But Neeva’s expertise and technology is clearly starting to flash some of its potential in one of AI’s biggest emerging solutions for hallucinations: an incredibly deep level of expertise in retrieval augmented generation (or RAG). Neeva in particular was a very early AI startup (like, January early) to deploy RAG at an enormous scale as it tried to build a new search engine, and that kind of architecture seems uniquely suited for a sprawling ecosystem that Snowflake seeks to offer its customers.
While OpenAI’s GPT-series and Anthropic’s Claude-series models make sense for a lot of use cases, the biggest appeal to enterprises with these tools is the ability to index and retrieve results using proprietary data—the kind of data that lives in Snowflake in the first place. And those same enterprises are still obviously quite skittish about shipping it over to OpenAI, regardless of the promises they make about security and privacy.
The same isn’t necessary true about Snowflake (or Databricks), which has already won the trust of those enterprises to host data and make it accessible. And Snowflake stands to provide companies with a true serverless option to use language models with their data heavily augmented with an entire ecosystem built up over years on top of its data sharing paradigms.
“Customers have already entrusted us with the data, which sometimes is trusting in eight quarters worth of scrutiny and regulatory assessment, or pen-testing, or other ways,” Snowflake chief product officer Christian Kleinerman told me. “They run us through our paces, and sometimes it takes even more than two years. But now that you have the data, and I offer a generative AI model or LLM on your data, I can vouch and guarantee the data is not copied out.”
Of course, Databricks faces the same uphill battles to get corporate data, and in the same way can potentially secure that level of trust and capability to power AI use cases within enterprises. Snowflake and its primary not-quite-but-sort-of rival have skirmished with one another in a variety of fields—with modern AI now becoming its biggest flashpoint yet.
An ongoing duel with Databricks
Indeed, the two seem to both be converging on the deployment of smaller, more customized models (chaotically referred to as fine-tuned, though there’s some nuance) that are much cheaper and faster to inference. They come at it from slightly different angles, but the end result is the same: get AI in production at scale, cheaply, and without the annoying rate limits companies run into with OpenAI’s GPT-series models.