Context windows and GPT-4's response quality
Two papers circulating this month highlight a new challenge we face working with LLMs: how sensitive they are.
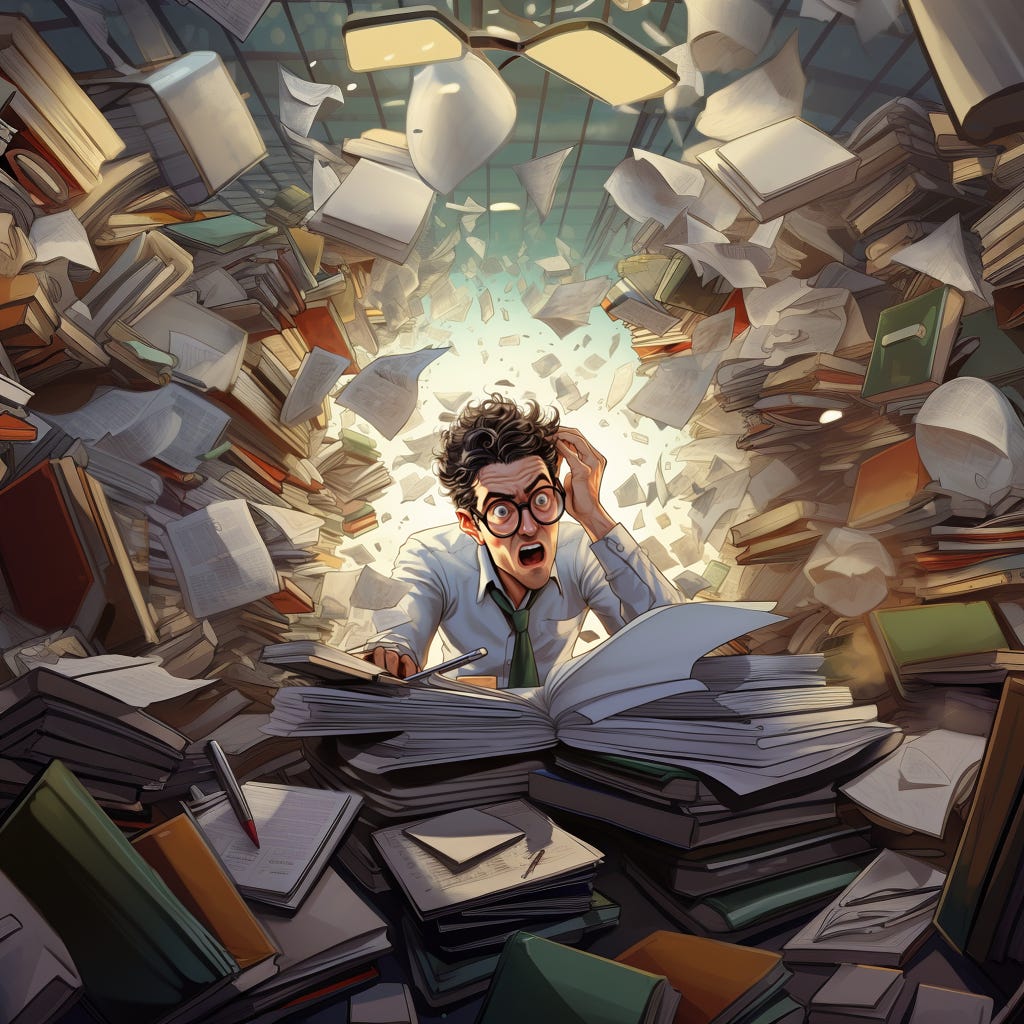
Today’s issue will be a little different as I want to take a look at two major themes that have been going around for the past two weeks or so.
One is the “lost in context” paper that came out of Stanford which was very much the topic du jour at the Pinecone Summit last week. The paper details how stuffing as much information into a context window might not yield the intended results—at a time when there’s an obsession with increasing the length of context windows.
The second is what we’ll call The Discourse around the “dumbening” of GPT-4. We had a fun moment this week where experts addressed a pet theory floating around the internet that GPT-4 was becoming less performant over time, and the reality is much more complicated.
While they feel separate, they’re both relatively related in so far as they touch on the sensitivity—and ever-changing nature—of these language models. And, more specifically, how we’re frantically trying to keep up with the technology as it changes rapidly.