An AI investor dilemma on the horizon
The tsunami of new AI startups targeting each shard of the LLM deployment stack presents a new question: how to invest without competing with older portfolio companies.
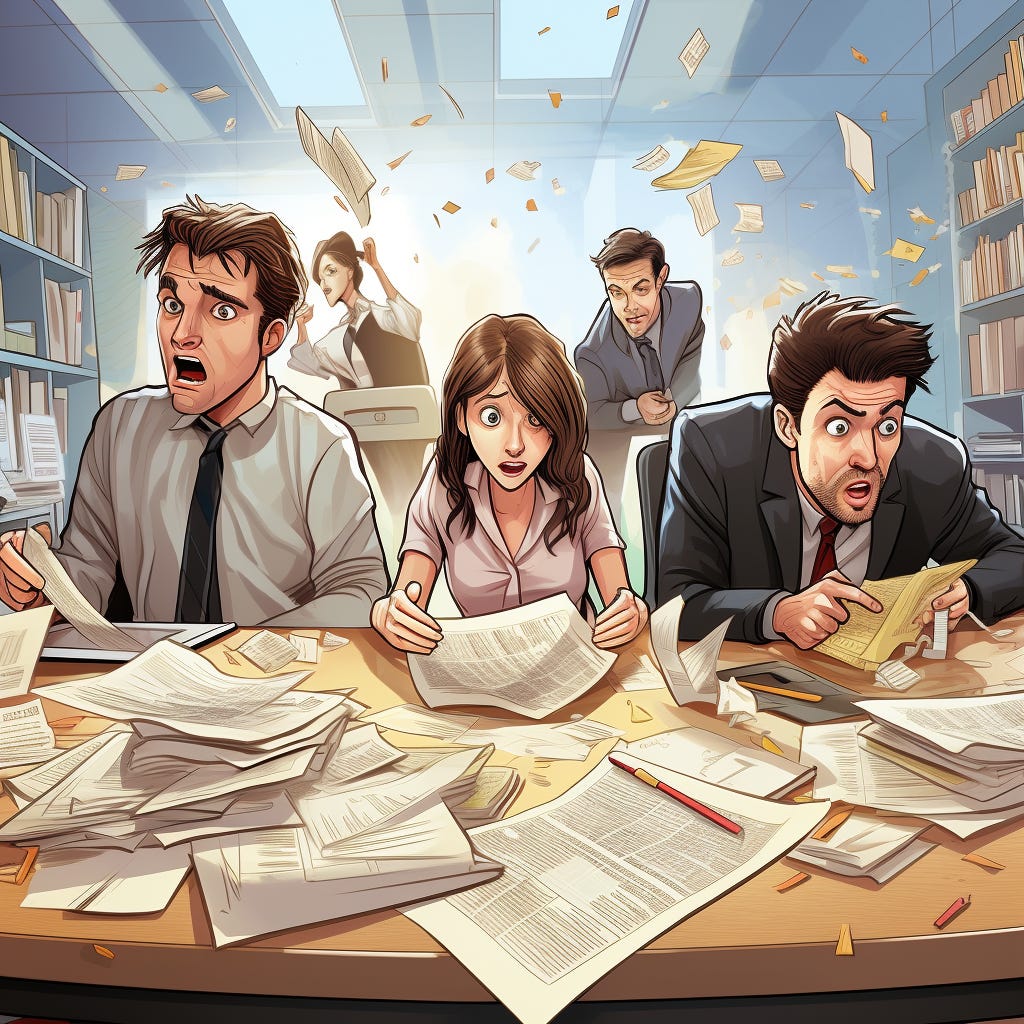
We’ll be covering two topics today! First on how investors have to find ways to ride the NEW AI hype wave when they already rode the last one; then, OpenAI’s acquihire and what the team actually brings to the table for its consumer product future.
A new emerging question for AI investors: competing with earlier portfolio companies
Generally speaking, when you talk to investors, they acknowledge the challenging funding climate but leave a small caveat at the end of the sentence for AI.
AI has created a level of hype that comes in shooting distance of the early iPhone, mostly because it sorta works in some cases and it’s cool as hell. We can debate whether or not it’ll end up being an extraordinarily successful industry or just a niche, but for the time being it has the hearts and minds of most of the internet-connected universe.
But coming with that tsunami of startups and ideas attacking nearly every part of the AI stack, there’s another dilemma emerging among investors: avoiding competition with existing AI portfolio companies that started int he last AI hype cycle (call it 2019-2022). In particular, portfolio startups have grown to encompass a larger portion of the machine learning stack will inevitably compete with a new post-ChatGPT generation trying to pick off anything that remotely touches an LLM or diffusion model.
I’m (unfortunately) old enough to remember one of the first inside baseball dust-ups in this vein: Andreessen-Horowitz’s sorta-colliding investments of PicPlz and Instagram (technically, Burbn at the time). It happened at pretty much the apex of the iPhone hype cycle, and one obviously did better than the other.
The whole timeline is extremely wonky for it, but the fact is it created a Weird Moment in the highest points of the Web 2.0 boom. It also highlighted a general house rule in venture investing: avoid—or at least do your best to avoid—investing in companies that have competing products or businesses.
The earliest days of the machine learning hype cycle saw the the birth of some more successful companies today like Weights & Biases, Hugging Face, MosaicML, and such that all started by attacking some shard of the machine learning stack. We got one for feature stores (Tecton), one for experiment tracking (W&B), a GitHub for open source (Hugging Face), AI training frameworks and tools (AnyScale), so on and so forth.