32 wild guesses for what happens in AI in 2024
I have to do this, apparently, like everyone else. So here are some shots in the dark as to what might happen for a field that's shown it's impossible to predict.
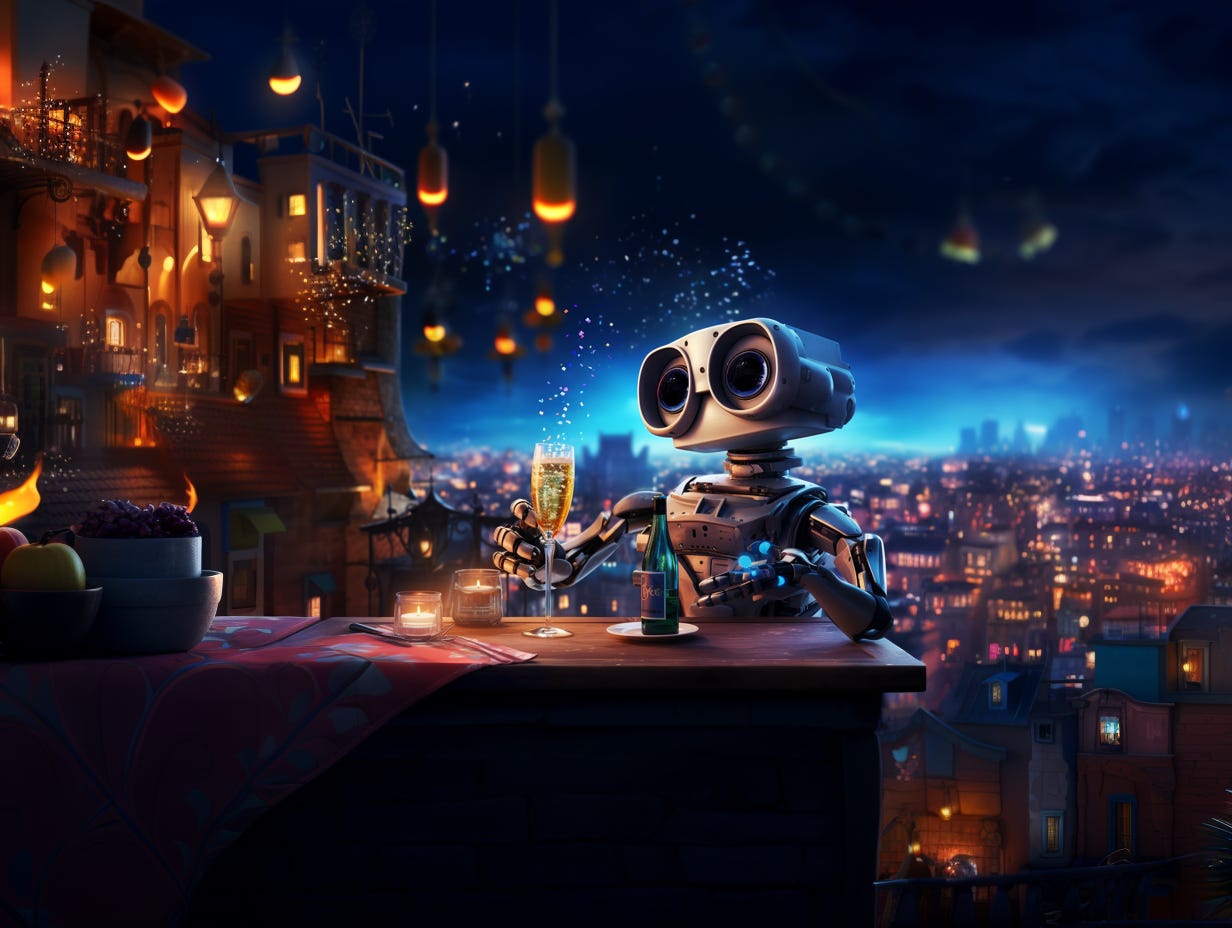
Author’s note: Since everyone has indeed logged off this week, tomorrow’s issue will be moved to the first week of the new year, so there will be three to kick off 2024. See y’all in a few days!
Alright, we’re doing this!
One of the most bizarre and exciting years in recent memory was defined the proliferation of an industry-altering technology—actually working AI. We started with the release of ChatGPT (last year, technically), then the first Llama model from Meta leaking onto the web, followed shortly by the launch of GPT-4. OpenAI has cemented itself as the current de-facto leader amongst the foundation model providers, with some challengers approaching.
Amongst all that we got *deep breath* the release of Mistral and its powerful successor Mixtral, OpenAI’s consumer tooling around assistants and GPTs, a smorgasbord of other APIs from providers like Cohere and Anthropic, open source technology actually catching up via startups serving them like Together and Anyscale, Databricks acquiring MosaicML for $1.3 billion, Snowflake making possibly the first true splashy acquihire of Neeva, benchmarking becoming a thing and a thing again, Google announcing but not releasing Gemini Ultra, Sam Altman briefly getting kicked out the building until everyone threatened to quit, a bunch of companies going after embeddings, LangChain becoming a business, a bunch of weird techniques popping up like quantization, Nvidia doing stuff, and so on.
If you skipped over that wall of text—and I honestly hope you did—I definitely do not blame you. As far as years in tech go, this has been one of the most chaotic and fun ones going all the way back to 2010. (I’d love to know if it was like this during the dotcom boom too.)
2023 is also a wrap in the next few days, after what feels like an eternity. And as is customary for any publication slash newsletter slash whatever, it seems I’ve been put in a position where I have to predict what’ll happen next year in AI. Because that’s completely possible to do and I will most certainly be completely accurate.
So in the spirit of 2023 being one of the most bonkers years in memory in tech, rather than do a bunch of big-headline-capital-P-predictions, I’m just going to throw out a bunch of wild guesses as to what developments might happen in 2024. This list is going to cover a lot of ground, including OpenAI, open source models, benchmarks, startups, Apple, more startups, and so on and so forth.
Based on how this year went, I’m going to generously guess I’ll be somewhere around 10% to 20% accurate. But hopefully I’ll get a B+ for effort here. And, as usual, this list is nowhere close to exhaustive.
With that, let’s get to it!
My “predictions” for what happens in AI next year
OpenAI revisits GPT-3.5 Turbo: With the release of the Mixtral MoE model from Mistral AI, OpenAI is losing the price battle for a highly competitive model with some serving companies offering inference as low as $.50 per million tokens. GPT-3.5 Turbo is OpenAI’s bread-and-butter model, and it will probably be looking at updates to improve its performance and gain at least a slight edge while reducing its cost substantially.
The foundation model providers release a standard benchmark: After a year of opaque data and benchmark gaming to make one look better than the other, Google, OpenAI, and friends are converging in mega-model performance. As a result, I believe one or more of them will put their heads together and come out with a unified benchmarking framework that shows “hey, we’re all still better than open source models,” while leaning into the story that open source being an advanced (and difficult to implement) alternative for niche use cases.
Embedding performance spikes, and the major data layers jump on board: We’ll probably see embeddings become a very important market for some of the API providers like Cohere and OpenAI as RAG becomes a permanent part of modern apps. And I expect some of the larger data layers—like MongoDB—to eventually get into embeddings.
Local models become a permanent fixture: The mixture of experts approach has basically been proven out, thanks to Mistral, as a viable path forward for getting performant models running on edge devices. Look for more MoE-type models that are designed to run on laptops, phones, and other devices.
Vector databases stick around, but get more specialized: As much as MongoDB and others would love you to think the majority of use cases wouldn’t need a vector database, it’s underselling how big the need is going to be. I think more companies are going to be shifting to a more aggressive micro-batch updating system where embeddings happen more frequently for better RAG. The touch points there are the embedding model and the vector database itself, and both are areas where you can pick up additional performance. However…
PGVector becomes the “good enough for most” option: Postgres is used literally everywhere. Everywhere. And amongst all the conversations I’ve had with developers, a lot of attention is going back to PGVector. Look for Neon, Supabase, and other associated Postgres-centric startups to be really important going forward here as PGvector provides a really seamless path toward creating a vector database for even more casual companies.